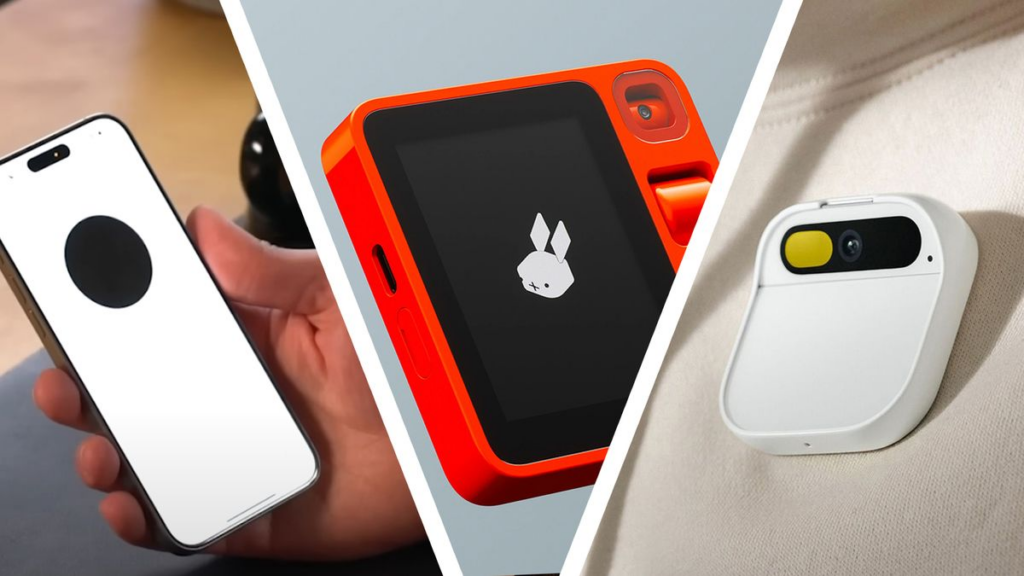
In the current era of personalised healthcare, there is an increasing need to integrate consumer health technology products to empower clinicians and patients. The growing ubiquity of wearable devices is evident, with an estimated 15%-25% of Canadians and more than 25% of those in the United States owning a device that tracks fitness or monitors health (1).
This translates into over 72 million US users within the past year alone. The global market for smart wearables is projected to reach CAD$70 billion by 2025, underscoring the vast potential for harnessing these data and the significant potential for improving health (2).
Wearable technology refers to devices designed for use while worn, with the primary function of measuring and recording physical parameters such as step count, heart rate and blood oxygen levels (3). The range of these devices extends from smartwatches to subcutaneous sensors, which are capable of sensing, recording, storing and transmitting data using various sensors.
These sensors may include barometers, accelerometers and magnetometers. The utilisation of these sensors enables the monitoring of health-related metrics, such as heart rate, blood pressure, oxygen saturation, body temperature, sleep, physical activity level, electrocardiogram (ECG), and biochemical parameters (e.g. serum glucose level).
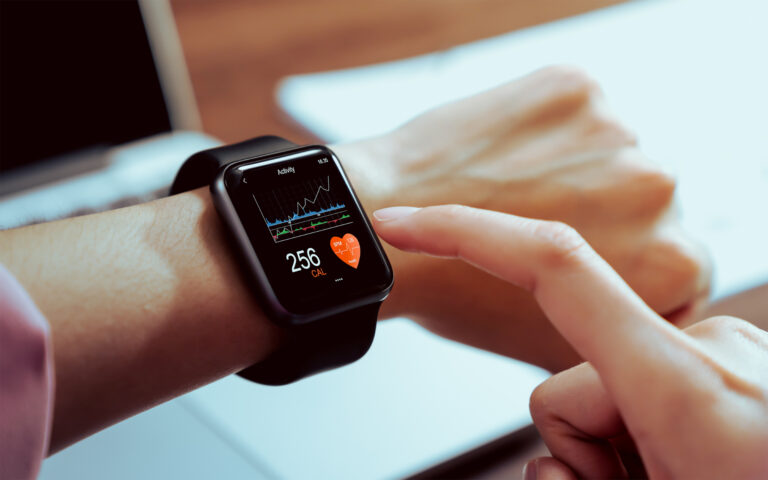
Physiological data obtained from wearables are of limited clinical utility when viewed in isolation. Indeed, clinicians have historically reported concerns with providing timely and accurate interpretation of these data (4,5). In this context, advancements in artificial intelligence (AI) have the potential to unlock this wealth of wearable data alongside clinical, laboratory and imaging modalities (6).
From Stethoscope to Software: Machine Learning’s Cardiac Revolution
Machine learning is a set of computational techniques that can identify intricate patterns within vast data sets, and subsequently produce precise and customised outputs. In the domain of cardiovascular health, there has been a historical focus on rules-based algorithms and probabilistic models for clinical decision-making. To illustrate this point, one may consider a blood pressure remote monitoring platform that would suggest an increase in the dosage of an antihypertensive medication based on preset thresholds.
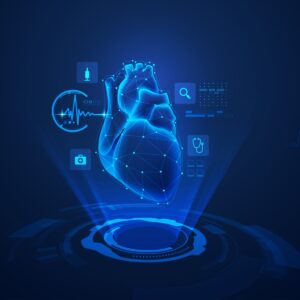
In recent years, there has been a growing trend in cardiology toward the adoption of deep learning, a subset of machine learning algorithms. They use neural networks to analyse and extract complex patterns from high-dimensional data such as electrocardiograms (ECG), echocardiography videos, textual reports, and medical images.
Deep learning can be supervised, unsupervised, and self-supervised learning. The supervised learning paradigm, one of the most widely used in cardiology, relies on labelled datasets where each input (e.g., an ECG waveform) is associated with a known output (e.g., a diagnosis). The model learns by making predictions, comparing them to the true labels, and adjusting its parameters based on the discrepancy (loss function) between predicted and actual outcomes. Repeated optimization allows deep learning models learn to recognize patterns in raw medical data, enabling more accurate predictions and diagnostic insights.
Deep neural networks (DNNs) have become a significant tool for cardiology, with applications in acquiring features and predictions from raw data (7). DNNs consist of intricate artificial neural networks comprising multiple layers of input and output, which are used to manage unlabelled and unstructured data. Their ability to extract features from a wide range of high-dimensional data sources enables data-driven discoveries, a crucial step forward in the field.
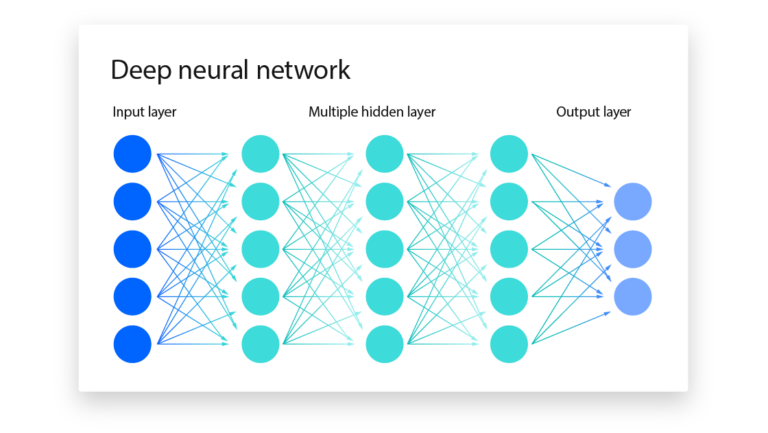
Current instances of these clinical applications include the utilisation of electrocardiograms (ECGs) to predict left ventricular dysfunction or future incidence of atrial fibrillation (AF) (8-11). However, it must be noted that the conventional approaches currently employed in this field are limited in terms of scalability and adaptability.
The integration of wearable data with artificial intelligence has emerged as a pivotal opportunity, particularly for the development of deep learning models. These models can encompass diverse sensor parameters, including but not limited to cuffless blood pressure, heart rate, oxygen saturation, temperature, activity, and blood glucose levels. The widespread utilisation of wearable sensors, in conjunction with the absence of enhanced data aggregation capabilities, has given rise to novel challenges in the management, interpretation, and integration of such data. The address of these challenges necessitates the development of multimodal artificial intelligence solutions that are capable of capturing the complexity of health and disease (12).
While integration of AI into medical care has been limited, progress has been seen in the application of medical imaging interpretation. However, given that clinicians routinely handle data from multiple sources and modalities, there is a pressing need to develop AI models that effectively integrate multimodal data.
Trusting the Machine: The Ethical and Logistical Challenges of AI in Medicine
The use of software algorithms that use artificial intelligence to analyse data collected by sensors has the capacity to process and collect vast amounts of data. However, challenges related to data privacy, usability and integrity are impeding the scale-up of wearable devices in clinical medicine. In addition, there is a need for rigorous, ongoing evaluation of these algorithms to determine their generalisability and feasibility of implementation in prospective data, clinical trials and feasibility studies to improve workflow.
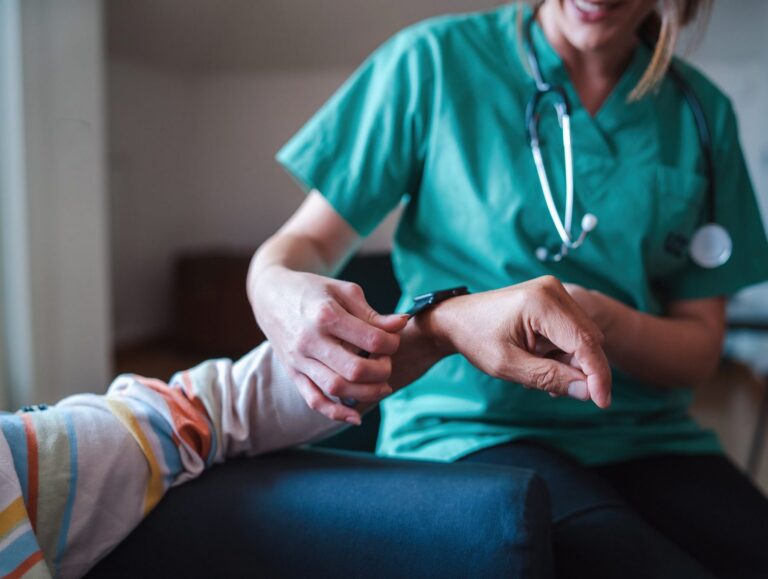
AI-based analysis of data from multiple sources has the potential for effective prevention and management of cardiovascular disease, but large-scale clinical trials are needed before it can be used. This transition presents a unique set of challenges, especially in ensuring the accuracy and validity of the biosensors in these devices. Indeed, the integration of wearable technologies requires the active involvement of clinicians and patients to ensure that these products meet their needs, are easy to use and inspire confidence.
The establishment of the necessary infrastructure is essential to facilitate decentralised healthcare delivery and ensure equitable access to wearable technologies and associated AI algorithms. In the future, healthcare providers will gain valuable insights into an individual’s cardiovascular health and deliver personalised care interventions by using real-time, patient-generated data from free-living environments.
Bibliography
- Shandhi MMH, Singh K, Janson N, et al. Assessment of ownership of smart devices and the acceptability of digital health data sharing. NPJ Digit Med 2024;7:44.
- Dagher L, Shi H, Zhao Y, Marrouche NF. Wearables in cardiology: here to stay. Heart Rhythm 2020;17:889-95.
- Iqbal MH, Aydin A, Brunckhorst O, Dasgupta P, Ahmed K. A review of wearable technology in medicine. J R Soc Med 2016;109:372-80.
- Yetisen AK, Martinez-Hurtado JL, Ünal B, Khademhosseini A, Butt H. Wearables in medicine. Adv Mater 2018;30:e1706910.
- Patel S, Park H, Bonato P, Chan L, Rodgers M. A review of wearable sensors and systems with application in rehabilitation. J Neuroeng Rehabil 2012;9:21.
- Brahmbhatt DH, Ross HJ, Moayedi Y. Digital technology application for improved responses to health care challenges: lessons learned from COVID-19. Can J Cardiol 2022;38:279-91.
- Perez MV, Mahaffey KW, Hedlin H, et al. Large-scale assessment of a smartwatch to identify atrial fibrillation. N Engl J Med 2019;381:1909-17.
- Raghunath S, Pfeifer JM, Ulloa-Cerna AE, et al. Deep neural networks can predict new-onset atrial fibrillation from the 12-lead ECG and help identify those at risk of atrial fibrillation-related stroke. Circulation 2021;143:1287-98.
- Raghunath S, Ulloa Cerna AE, Jing L, et al. Prediction of mortality from 12-lead electrocardiogram voltage data using a deep neural network. Nat Med 2020;26:886-91.
- Noseworthy PA, Attia ZI, Behnken EM, et al. Artificial intelligence guided screening for atrial fibrillation using electrocardiogram during sinus rhythm: a prospective non-randomised interventional trial. Lancet 2022;400:1206-12.
- Attia ZI, Kapa S, Lopez-Jimenez F, et al. Screening for cardiac contractile dysfunction using an artificial intelligence-enabled electrocardiogram. Nat Med 2019;25:70-4.
- Acosta JN, Falcone GJ, Rajpurkar P, Topol EJ. Multimodal biomedical AI. Nat Med 2022;28:1773-84.
- Laura Avogaro from FRESCI
- No Comments